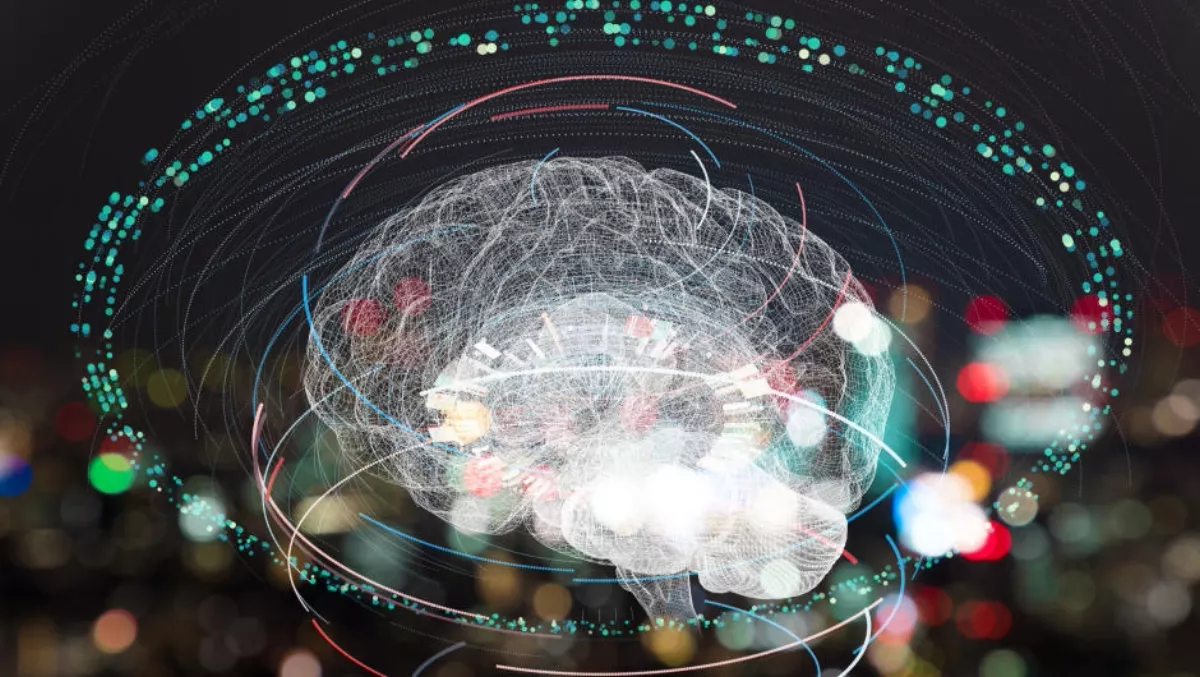
Artificial intelligence oversaturation – going beyond the buzzword
As businesses are storing more data than ever before, the need to better manage and benefit from big data is resulting in massive growth in artificial intelligence (AI) technologies being applied more broadly across industries and applications. Unfortunately, emerging technologies have a habit of becoming marketing jargon and artificial intelligence is one of the industry's most overused buzzwords right now.
Cybersecurity is one such industry that is in the midst of AI oversaturation, with start-ups and vendors associating AI with their products and in some instances wrongly labelling their solutions as "AI-driven" or "based on AI" without truly leveraging the full power or promise of the technology. This oversaturation is confusing the marketplace and its buyers, and can lead to a wrong sense of security in certain instances.
Adding to this confusion is the interchanging of machine learning (ML) and artificial intelligence.
The two terms are used interchangeably quite often, but ML is one component of AI. What vendors really mean when they talk about AI is what professionals in the field call ML.
The reality is that ML in cybersecurity can be applied in many ways; some solutions use these techniques entirely to identify new threats, whereas other solutions use it as a way to identify new user behaviours. Some more traditional security tools are trying to adapt AI concepts to try to overcome gaps in signature detection techniques.
This over-hype will mean that a number of solutions will ultimately fail, or more specifically not deliver the claimed silver bullet and the end-user is the one that will suffer. With so much confusion, enterprises need to know how to cut through the hype and identify what true value is, rather than just marketing talk.
ML in cybersecurity
One very important role of ML in cybersecurity is to detect new and unknown threats in real time. The sheer number of attacks seen on a daily basis is beyond the capability of traditional security based detection and many attacks in 2017 devastated organisations simply because of failed detections. In CrowdStrike's recent global threat report, it identified that 39% of all detections in 2017 were malicious software than went undetected by traditional AV.
As cybersecurity companies talk about prevention in terms of detecting behaviour rather than looking for every attack based on a signature or a pattern match, technology development needs to take into consideration massive data sets, the algorithms to leverage the data, and importantly the implementation of the technology within a countermeasure solution. Ultimately, the product needs to detect unknown malware with fewer false positives.
As detecting never-before-seen threats is not a trivial task, a company's ability to build a broad platform to collect massive and diverse threat data sets is paramount. Organisations that fail to build this platform will face a high bar of entry into true ML use in cybersecurity as ML functionality is only as good as the data input that trains the algorithms.
Dispelling market hype
The recent explosion of ML capabilities has been fuelled by decades of improvements in computing power, combined with progress in algorithms and an increase in the volume of data. With the market flooding with ML solutions, it can be difficult to tell them apart and the implication is a mix of confusion, noise, and ineffectiveness.
As more solutions crowd the market touting the benefits of ML, it is important to identify and read through the market hype. While what ML has to offer is impressive, as with any technology, there are limitations, and there are certain security issues that are best solved by other techniques. The reality of large breaches is that attacker works for months, if an adversary targeting your organisation is persistent enough, they will eventually get a piece of malware past even the best ML-based defences.
While improving the ability to detect new threats, ML alone does not simply fix the cybersecurity challenge. Having comprehensive endpoint protection – that includes ML but also offers exploit prevention and behavioural analysis – should be an integral part of the solution you choose. The ability to detect subtle signs of an attack, based on analysing event behaviours and determining an attacker's intent, is also a critical function that provides protection regardless of the malware or exploit used in the attack.
Understanding that attackers will be creative, technology alone cannot prevent every attack. For file based attacks to succeed, a persistent threat actor only needs to be able to execute a single file in your environment. When looking at efficacy of solutions, consider the statistic of 99% threat detection.
If an adversary builds 100 malware attacks and tries to get them into your environment, there is potential that one will get through. If the adversary replicates the approach of sending 100 malware attacks, but this time attempts to do this 500 times, there is potential for 500 attacks to get through. While still a 99% detection rate, the target of the attacker has had to defend against a high volume of attempted attacks.
This increased attack at volume, together with the fact that more than half of the attacks from a sophisticated adversary are not file based, requires a multi-factor approach. It is important to look at ML together with other techniques such as exploit prevention and behavioural analysis when looking at the best architecture for endpoint security as an example.
Being able to analyse behaviours and importantly being able to analyse an attacker's intent is an important function of any security architecture. An effective next-generation endpoint architecture must also be supported by a team of security experts hunting through available data and proactively looking for threats.
Being able to learn from incidents that have taken place, leveraging aggregated data, analysing it and providing response guidelines when malicious activity is discovered, is the value delivered by a team of threat hunters.
The oversaturation of labelling AI and ML in cybersecurity cannot be overstated. They're both vital in the fight against today's threats, but only those organisations that use them effectively – not in isolation – will rise to the top to truly improve their defence against threats.