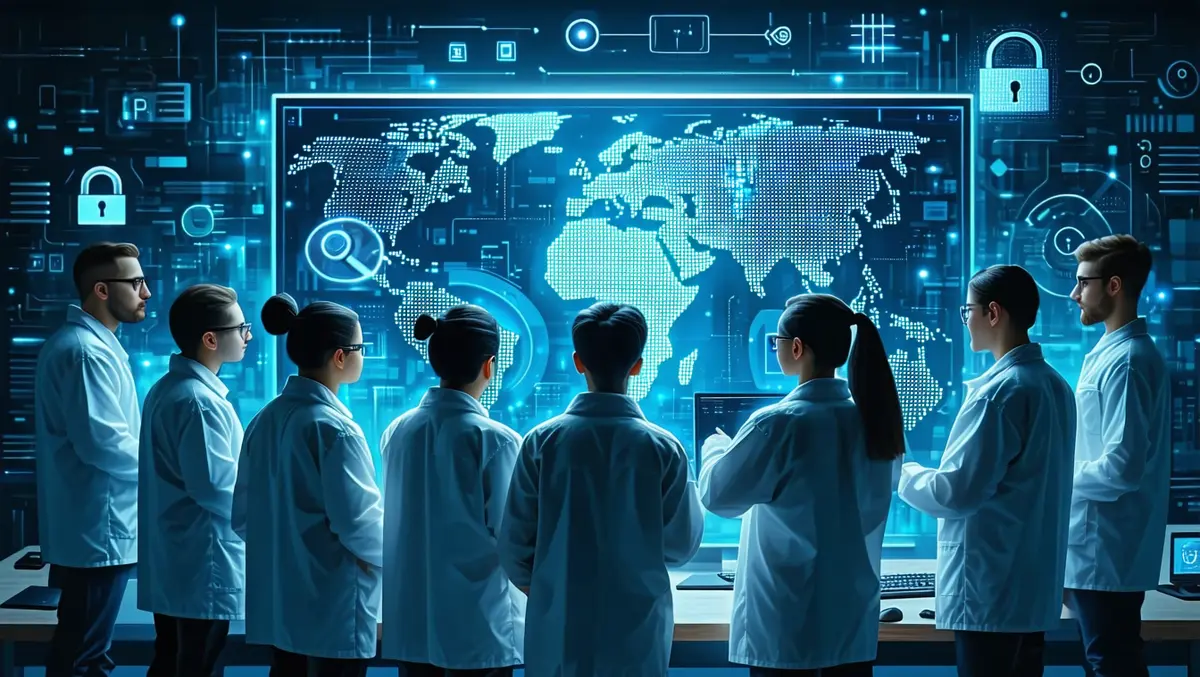
Open-source AI Foundation launched to boost transparency
The Open-Source AI Foundation has been launched with the mission to enhance transparency and accountability in AI systems used by civilian government agencies, coinciding with DeepSeek's announcement to open source some of its AI models' code.
Andrew Stiefel, Senior Product Marketing Manager at Endor Labs, discussed the significance of applying transparency practices to AI systems, drawing parallels with the U.S. government's 2021 Executive Order on Improving America's Cybersecurity. He said, "The U.S. government's 2021 Executive Order on Improving America's Cybersecurity includes a provision requiring organizations to produce a software bill of materials (SBOM) for each product sold to federal government agencies. This provides a full inventory of the open-source components used within the product, and helps the Federal government track vulnerabilities that could impact government systems. Applying these same principles to AI systems is the logical next step. This provides better transparency for citizens and government employees, but also improves security by giving visibility into a model's datasets, training, weights, and other components."
Stiefel also expressed support for DeepSeek's decision to open its models' code, having labelled the move as beneficial in terms of transparency and security. "DeepSeek has already released the models and their weights as open-source. This next move will provide greater transparency into their hosted services, and will give visibility into how they finetune and run these models in production. This will make it easier for the community to audit their systems for security risks, and also for individuals and organizations to run their own versions of DeepSeek in production," he remarked.
He further highlighted the importance of understanding providers' operational approaches: "From a transparency side, we'll see how DeepSeek is running their hosted services. This will help address some of the security concerns that emerged after it was discovered they left some of their Clickhouse databases unsecured." The move could potentially set a precedent for other AI companies in terms of demonstrating their operational protocols.
Julien Sobrier, Senior Product Manager at Endor Labs, commented on the need for a clear definition of "open" in the context of AI models. Sobrier noted, "An AI model is made of many components: The training set, the weights, and programs to train and test the model, etc. It is important to make the whole chain available as open source to call the model 'open'. It is a broad definition for now. Among the main players, the concerns about the definition of "open" started with OpenAI, and Meta is in the news now for their LLAMA model even though that's "more open". We need a common understanding of what an open model means. We want to watch out for any "open-washing" as we saw it with free vs. open source software."
Regarding the shift in open source projects, Sobrier remarked on the trend towards commercial restrictions: "With cloud providers offering a paid version of open source projects (such as databases) without contributing back, we've seen a shift in many open source projects: The source code is still open, but they added many commercial restrictions. Meta and other "open" LLM providers might go this route to keep their competitive advantage: more openness about the models, but preventing competitors from using them."
Both experts emphasised the growing intersection of AI model deployment and a systematic risk management approach. Stiefel observed, "This rapid adoption of open source AI models highlights a crucial reality: Organizations need a systematic approach to evaluating and managing AI model risk. While open source models aren't inherently more risky than proprietary ones, they do require careful evaluation and ongoing monitoring."
Sobrier underscored the need for companies to adopt best practices in ensuring the safety of AI models, saying, "We need to look at AI models as major dependencies that our software depends on. Companies need to ensure they are legally allowed to use these models, but also that they are safe to use in terms of operational risks and supply chain risks, just like open-source libraries. They need to be confident that the datasets used for training the LLM were not poisoned or had sensitive private information. The community needs to build best practices to develop safe and open AI models, and a methodology to rate them along security, quality, operational risks and openness."